This blog is based on the breakout session titled “Responsible Human-Machine Teaming Across the AI Lifecycle: An Interactive Scenario”, co-organised by the AutoNorms project and Johns Hopkins University Applied Physics Laboratory (JHU APL) at the Responsible AI in the Military Domain (REAIM) Summit 2024 in Seoul, South Korea. You can watch the breakout session here.
Autonomous and AI technologies are increasingly integrated into weapon systems and military decision-making processes, as seen with the use of decision support systems in the ongoing Israel-Hamas war. Some argue that the employment of such systems represents a form of human-machine interaction or human-machine teaming because machines are not intended to replace humans by design. However, several critical questions in relation to military applications of AI remain highly debatable. To what extent can humans effectively exercise their agency in their interactions with AI systems? Do the uses of these systems introduce complexities that might hinder or complicate the process of exercising human agency? And even if the development and application of these systems are considered an ‘unavoidable’ trend, the trajectory of this development is by no means clear and needs to be shaped in ‘responsible’ ways.
This blog takes inspiration from the discussion at the REAIM 2024 breakout session on “Responsible Human-Machine Teaming Across the AI Lifecycle” that the AutoNorms team co-organised with the JHU APL. The blog argues that although humans currently remain ‘in’ or ‘on’ the loop of decisions integrating AI-based systems, these human-machine interactions involve significant challenges in exercising human agency, broadly defined as “the ability to make choices and act“, in the military domain, especially in the use of force. To illustrate this point, the blog examines three concerns that affect humans’ potential to exercise qualitatively high levels of agency in human-machine interaction. These are the uncertainty and complexity of ‘contexts’, the trade-off between efficiency and effectiveness, and the challenge of balancing speed with transparency.
Uncertainty and complexity of ‘contexts’
The first challenge to the effective exercise of human agency is the uncertainty and complexity of ‘contexts’, which can be viewed from two perspectives. The first is the context in which AI-based systems are developed, deployed, and used. As Lt Gen John (Jack) Shanahan (USAF, retired) highlighted, no matter how rigorously AI systems are trained and tested, it is impossible to fully replicate the conditions of an operational environment. The unpredictability of real-world warfare and battlefield scenarios, which often differ from the controlled conditions of training, can cause AI systems to behave unexpectedly or malfunction. This uncertainty makes it more difficult for humans to fully grasp the context and make informed decisions, especially when end users lack sufficient knowledge of the systems’ underlying mechanisms. Additionally, Shanahan highlighted that acceptable levels of risk and tolerance for errors are context-sensitive. In high-intensity combat, decision-makers may accept higher levels of risk due to the urgency and speed of operations. In such complex situations human operators may struggle to exercise a qualitatively high level of agency, making it difficult to identify, report, or correct failures in AI systems’ outputs.
The second aspect, highlighted by Leverhulme Early Career Fellow Dr Tom Watts, concerns the broader ‘context’ of geopolitical competition. Great power rivalry and geopolitical considerations influence how individuals and states exercise their agency and judgement, as well as how they delegate tasks once performed by humans to machines. This is because perceptions of the effectiveness and strategic importance of AI systems in the military are shaped by how states believe their adversaries or competitors will value and use these technologies. This is particularly relevant in the US-China tech war, where fears of one side gaining superiority in AI-based military technology can push the other side to adopt more aggressive strategies. This could result in reduced levels of human agency and increased autonomy in decision support and weapon systems, potentially establishing such choices as shared values in the military domain.
To capture the importance of ‘context’ in the exercise of human agency, some suggest using the term “context-appropriate human involvement” in addition to the more widely used ‘meaningful human control’. This concept accounts for contextual factors such as “the purpose of use, the physical or digital environment, and the nature of potential threats”. Nevertheless, it remains unclear how to achieve such appropriate human involvement in practice, given the significant uncertainty and complexity of real-world contexts, particularly in light of the speed at which these systems operate.
Efficiency versus effectiveness
AI-based systems provide opportunities to enhance the efficiency of human decision-making and action, potentially enabling military objectives to be achieved more quickly and cost-effectively. However, concerns have emerged about whether this increased efficiency might come at the expense of effectiveness. Between the two values, efficiency focuses on achieving a goal with minimal waste of resources such as time and costs, particularly for one’s own side. Effectiveness, on the other hand, focuses on how successfully the desired or intended outcome is achieved, not only in strategic terms but also in ethical and legal terms.
The potential for AI systems to accelerate command and control processes, gain strategic advantage, and enable faster action on the battlefield compared to adversaries is driving militaries to adopt these technologies. However, while AI-based systems are designed to reduce time-related costs, they introduce significant challenges, particularly concerning human agency. To optimise efficiency, these systems often compress the timeframe for humans to exercise their judgement and make informed decisions under intense time pressure. Human can become overly reliant on these technologies, such as trusting AI systems’ outputs without critically questioning those—a phenomenon known as automation bias. In AI-based decision support systems, the rapid speed of target generation and recommendations can create a sense of urgency, potentially pressuring human operators to make hasty decisions. This perceived urgency limits humans’ ability to thoroughly evaluate algorithmic recommendations and therefore restricts their opportunity to exercise a high level of agency. In such cases, humans risk becoming mere ‘rubber stamps’ in decision-making on the use of force, remaining symbolically ‘in’ the loop but potentially accepting recommendations without having the possibility to recognise or reject inappropriate ones.
Moreover, as Lynn Piaskowski, Supervisor of the JHU APL Human-Machine Engineering Group within the Air Missile Defense Sector, noted during the panel, “Machines make mistakes, too—just faster”. This issue is further exacerbated by the first concern: the uncertainty and complexity of real-world contexts. When the actual context differs from the scenarios in which the algorithms were trained and tested, their effectiveness decreases. This is also referred to as the problem of data brittleness, where AI algorithms struggle to adapt to new and uncertain environments. Therefore, a decision made faster with the help of an AI system does not necessarily give the edge to win a battle; it may simply lead to mistakes being made more quickly.
Regarding the trade-off between efficiency and effectiveness, the breakout session panellists provided a clear answer: effectiveness must take priority over efficiency. However, they also noted that in technical and military circles, there is often a push to automate systems to compensate for human limitations and to pursue efficiency in order to gain a strategic advantage through increased speed, reduced economic costs, and minimised casualties among their own forces during warfare. Since over-reliance on machines is considered inappropriate—given that machines are not perfect and can make mistakes—the key question becomes: how should ‘acceptable error rates’ for such systems be defined, if they should exist at all? This raises significant legal and ethical concerns about the consequences of these mistakes in real-world combat situations.
Speed versus transparency
The third concern stems from the challenge of balancing speed with transparency. As identified by Alexander Blanchard, Christopher Thomas, and Mariarosaria Taddeo in their recent article, transparency includes two types. First, there is system transparency, which refers to the openness of the elements of AI-based systems, such as data and algorithms. Second, there is systems development transparency, also known as traceability, which involves transparency in the research and innovation processes that produce AI systems. Of the two, systems development transparency carries greater ethical and legal significance, as it provides insight into decisions made throughout the AI system’s lifecycle—not only by end users, but also by those involved in the design and development phases.
Despite the ethical and legal importance of transparency, opinions are divided when it comes to striking the right balance between speed and transparency. Unlike the clearer consensus on prioritising effectiveness over efficiency, this division was evident in an audience poll during the REAIM breakout session, where participants were asked to choose between two options during the Research and Development stage of the AI lifecycle: (A) rigorous documentation with fully traceable accountability, chosen by 15 out of 29 respondents, and (B) flexible documentation with less stringent accountability, chosen by 14 out of 29 respondents (see the results below).
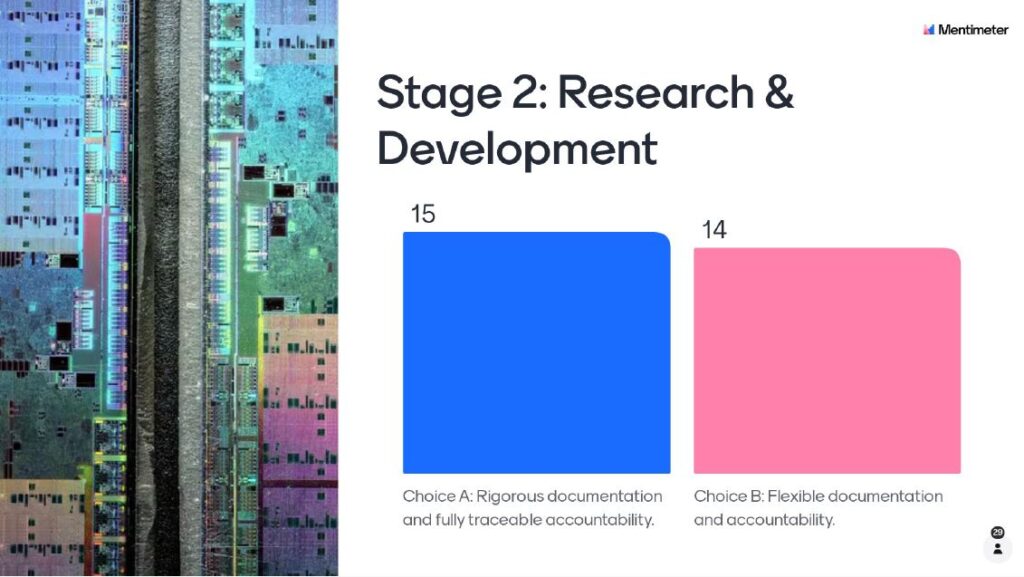
Commenting on the audience’s choice, Shimona Mohan, Associate Researcher at the UN Institute for Disarmament Research, emphasised that detailed documentation and transparency at the R&D stage are crucial for ensuring the exercise of human agency. She noted that documentation is not just about recording events, but also about understanding what is happening, consolidating that knowledge, codifying it into the system, and acting on it effectively. Nehal Bhuta, Professor of International Law at the University of Edinburgh, supported this view, adding that rigorous documentation has significant legal implications. It provides a record of uncertainties and trade-offs in testing, evaluation, validation and verification, offers essential evidence for evaluating whether actions meet legal requirements, and helps trace liability and accountability.
Piaskowski, on the other hand, highlighted the practical challenges of detailed documentation, noting that it can be difficult to manage and implement effectively. Shanahan echoed these concerns, questioning how much transparency can realistically be achieved in real-world combat situations, and whether detailed documentation would be useful in high-pressure scenarios, such as when an enemy aircraft is approaching at 500 mph. Thus, while transparency is critical, managing it—particularly given the fast pace of these systems and the realities of combat—presents significant practical challenges.
As Piaskowski critically asked: how can we maintain thorough documentation while still moving quickly to deploy capabilities? To address this question, she suggested involving end users from the outset and throughout the AI system’s lifecycle, including the prototyping phase. This approach allows users to understand the design as it evolves and learn the reasoning behind design decisions, enabling them to explore real-time conditions while simultaneously documenting the system.
While challenging to implement, especially in fast-moving contexts, transparency and documentation are crucial. From a technical and military standpoint, transparency can, to some extent though not always, enhance trust in these systems from both end users and the public, providing confidence that they are being used in a responsible and legitimate manner. From an ethical and legal perspective, transparency carries significant implications, providing the necessary foundation for accountability.
To address this, Zoe Porter and her colleagues propose a ‘good transparency’ model, which they suggest could serve as a standard for suitable transparency. This model is based on four elements: quantity (the right amount of information is communicated), quality (the information is truthful), relevance (the information is salient), and manner (the way information is conveyed promotes effective exchange and understanding). However, when choosing potential models of transparency and methods of documentation, a new set of trade-offs emerges—namely, between standardisation and adaptability. Should we adopt a standardised documentation framework, or opt for a more flexible, dynamic approach that tailors documentation to specific contexts, situations, or user groups?
Way forward
This blog explored three key aspects that highlight the complexity of exercising human agency in human-machine interaction across the AI lifecycle: the uncertainty and complexity of ‘contexts’, the trade-off between efficiency and effectiveness, and the challenge of balancing speed with transparency. Given these complexities, it is essential to move beyond the debate over whether humans are ‘in’ or ‘out’ of the loop. Instead, the focus should shift to the quality of human agency within the human-machine interaction, with particular attention to identifying the appropriate form of distributed agency between humans and machines in specific contexts.
To conduct this analysis effectively, it is also important to adopt a lifecycle approach—examining human-machine interaction not only in the end-stage of force application, such as in autonomous weapon systems, but across all stages, from research and development, procurement and acquisition, testing and evaluation, potential deployment, to post-use or retirement.
The author would like to express gratitude to Ingvild Bode and Anna Nadibaidze, whose valuable feedback greatly improved the analysis in this blog.